6 Ways Data Can Guide Learning
When educators use data effectively, it can lead to improvements in students’ learning and well-being.
Your content has been saved!
Go to My Saved Content.A few years back, one of us sat in a school district meeting where administrators and educators talked about the latest student achievement results. The news was not good. Students’ test scores hadn’t shown any significant improvements over the past year, and, in fact, some scores had gone down. After sharing the disappointing results, the district leader turned to the teachers who were attending and said, “Look. You have to teach better. Our students deserve better, and what you’ve always done isn’t good enough. Things have to change.”
The administrator’s desire to see improvement for students was well-intended, but the way he expressed it wasn’t helpful. Almost all the teachers in the room were dedicated to working hard for children. They wanted students to achieve as much or more than anyone in the room, but they didn’t know what they could do to increase their impact. If they knew what to change to reach more students, they would be doing it. The leader wanted to push teachers to improve, but most likely every teacher left the meeting unhappy, discouraged, and less open to change.
Meetings like this give data a bad name. Sharing low scores at the conclusion of the school year is discouraging for those who chose a career in teaching because they wanted to positively impact the lives of their students. But data don’t have to be used in this way. Used effectively, data can foster hope and lead to positive improvements in students’ learning and well-being. We’ve identified 10 Data Rules educators can use to guide their use of data. The first six rules are described below.
Rule #1: Data Should Foster Hope
University of Kansas researchers Rick Snyder () and Shane Lopez () have described three elements that are necessary for hope. People who have hope, the researchers have explained, have (a) a preferred future or goal; that is, something to hope for; (b) pathways to that goal; that is, some possible ways of meeting the goal; and (c) agency, a belief that they can hit the goal if they follow the pathway. When used correctly, data foster hope. Data can help teachers identify a preferred future by shining a light on particular aspects of student learning and behavior.
When teachers review data on students’ emotional engagement, for example, they will know more about their students’ current reality and they can use that knowledge to set goals related to students’ emotional engagement. Once teachers start making changes in their classroom to increase students’ engagement or achievement, they can gather data to monitor progress toward the goal. Used effectively, therefore, data help teachers (a) identify what they hope for, (b) measure the effectiveness of the pathway to the goal, and (c) build agency by showing what progress is being made. In short, data don’t have to feel like a punishment. Data can foster hope.
Rule #2: Data Should Be Tied to Professional Learning
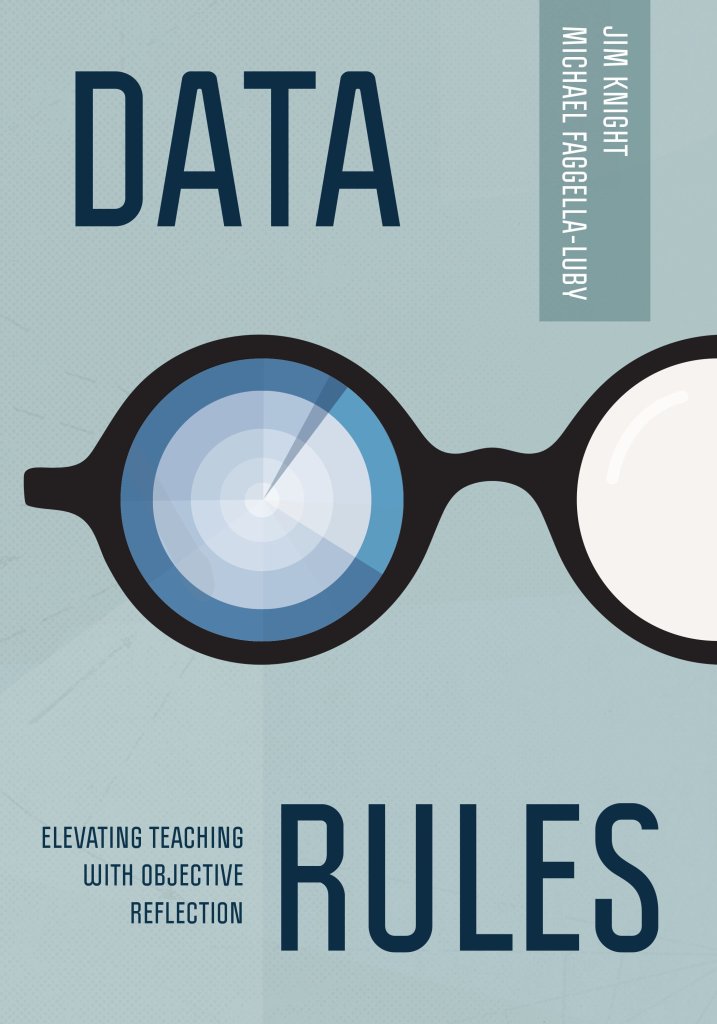
For teachers to have hope, they need to use data to identify a pathway that will enable them to move toward their goal, and then they need to use data to monitor progress until the goal is met. If teachers don’t see a pathway to their goal, they won’t feel hope. Professional learning helps teachers identify and follow pathways to their preferred futures. Often such learning takes place when educators partner with coaches to move through a coaching cycle. We have spent more than two decades developing, refining, and validating a coaching cycle we refer to as the Impact Cycle (). Although aspects of the Impact Cycle can be employed by teachers for self-reflection or by principals in partnership with teachers, the cycle is most frequently implemented by teachers with coaches. It consists of three stages—Identify, Learn, and Improve—and can be adapted for use by individuals, teams, or instructional coaches.
Rule #3: Data Should Be Chosen by the Teacher
There are many kinds of data that are not chosen by the teacher. State tests, for example, are outside the control of teachers and school leaders, and, like it or not, schools and teachers in the United States and other countries are often assessed by that data. Also, in most settings, teacher evaluation involves data that are not chosen by the teacher even though there might be great advantages to involving teachers in identifying the standards for evaluation.
When it comes to professional learning, however, there are many reasons why teachers should identify what data will be gathered to monitor progress to goals. First, each classroom is a distinct collection of a wide range of students guided by a unique teacher. Consequently, the most relevant data in one setting might not be as relevant and helpful in another setting. Also, the person who knows the most about the students and the teacher is, of course, the teacher. For these reasons, teachers are often best positioned to identify the data to be gathered for their professional learning.
A central assumption of this book is that teachers are professionals who use their professional judgment to make decisions about what happens in their classrooms. We talk about professional development (the learning activities teachers experience) and professional learning (the growth and transformation that is the result of effective professional development), but professional development and professional learning are only possible when we treat teachers as professionals. By telling teachers what their goals will be and what data will be covered, we are treating teachers more like workers on an assembly line than professionals.
Our students deserve professionals teaching them, but we won’t have professional teachers unless they have many opportunities to use their professional judgment.
Change happens from the inside out, not from the outside in. People are motivated by their own goals. If we want people to be motivated to change, we need to encourage them to set their own goals and identify their own data. Human beings are very skilled at nodding their heads yes and not changing, and if we want people to commit rather than comply, we are wise to encourage them to set their own goals.
A teacher, of course, may not know what data to gather. When this is the case, a coach who understands the kinds of data described in this book can be very helpful. A knowledgeable coach can suggest possible kinds of data that a teacher might choose. However, this should be a nuanced conversation. The coach should interact in a way that ensures that teachers choose the data while also clearly describing different types of data. A coach might ask a teacher for permission to share data, thereby demonstrating respect for the teacher. Then the coach could offer choices, saying something like, “When teachers have been interested in increasing engagement, they often look at increasing time on task, decreasing disruptions, or increasing instructional time by shortening how long transitions take or looking at how students respond to questions. Will any of these forms of data help you see if you are hitting your goal?”
Rule #4: Data Should Be Objective
Data can be gathered either objectively or subjectively. When something is subjective, according to the Oxford English Dictionary (OED; oed.com), it is “related to the thinking subject, proceeding from, or taking place within the subject, having its source in the mind.” Subjective data, then, are based on the thoughts in someone’s minds about what they are observing. When something is objective, on the other hand, it is based on something “external to the mind.” That is, objective data are “the actual facts, not colored by the feelings or opinions of the writer” (OED).
How we talk about data varies, therefore, depending on whether the data are subjective or objective. For example, someone who gathers subjective data might say, “You asked some good questions, but I don’t think your students were engaged.” In contrast, someone who gathers objective data might say, “You asked 17 closed questions and 4 open questions, and 5 of your 27 students answered the questions.”
A coach gathering objective data might even put tallies on a seating chart to show which students answered questions or note the cognitive level of each student’s responses. Objective data are the soul of providing authentic feedback that can be valued by all parties involved in the coaching conversation.
Used effectively, data can foster hope and lead to positive improvements in students’ learning and well-being.Michael faggella-luby and jim knight
There are several problems with using subjective data. First, our perceptions of reality are imperfectly filtered through our perceptual errors and defense mechanisms. If a teacher turns to an observer and says, “That’s not what happened in the class,” they usually are correct at least from their perspective.
Another problem with subjective data is that by virtue of their personal nature—they involve my opinion of what is happening in your classroom—subjective comments can trigger defensiveness on the part of the person receiving the feedback. When someone asks, “Would you like to hear my opinion on how you teach?” most of us, I suspect, if we were being really honest, would be inclined to say no. The reality is that our advice isn’t as good as we think, and people don’t want to hear it as much as we think (see Advice Trap [S] for an excellent discussion of the problems with the so-called Advice Giving Habit [AGH]).
The difference between subjective and objective data becomes clear if you watch the Winter Olympics. During speed skating, for example, when the race is over, the winner is the person who crosses the line the fastest. Assuming everyone is judged to have raced fairly, whoever makes it to the finish line in the shortest amount of time goes home with the gold. Consequently, there are very few controversies about who was the fastest during a speed skating race. This is how objective data work. There’s no opinion involved; the data just are what they are.
During figure skating, however, the data are subjective and the story, therefore, is completely different. Figure skaters, or at least figure skating commentators, often criticize the way in which skaters are scored. Indeed, the fact that gold medals are handed out based on subjective opinion has led to numerous arguments and controversies. During the 2002 Winter Olympics, in fact, Canadians Jamie Salé and David Pelletier were not awarded their gold medals until several days after the competition after many figure skating experts had criticized the subjective scores offered by judges.
Once selected, objective data are not personal—they are factual. When I gather and share reliable, objective data, my opinion doesn’t guide the conversation; I am just reporting the facts. In contrast, subjective data, by definition, always involve my opinion. Conversations about my opinion can turn the focus away from what happened in a classroom and toward whether or not my opinion is correct. For this reason, we suggest educators gather objective data. This book offers descriptions of dozens of examples of objective data anyone can gather.
Rule #5: Data Should Be Reliable and Mutually Understood
When researchers talk about reliable data, they mean that the data are consistent regardless of who gathers them. Thus, four different researchers observing the same classroom will produce the same results with little variation if their data gathering is reliable. This kind of reliability is also important outside of research situations, such as when administrators gather data for teacher evaluation. Most teachers would feel frustrated if two administrators gave them contrary evaluative feedback based on differing interpretations of a teacher-evaluation tool.
Reliability is a bit different in coaching situations. What matters there is that the coach and teacher have the same understanding of the data. For example, if a teacher wants to decrease the number of disruptions by increasing the ratio of interaction, it is important that the teacher trusts the coach’s data.
One way to increase mutual understanding is for the coach and teacher to create a T-chart that describes examples and nonexamples of data. For example, a T-chart for time on task might include “listening to teacher during direct instruction” on the example side of the chart and “engaging in side conversations” on the nonexample side.
Rule #6: Data Should Be Valid
Data are valid when they measure what they are intended to measure. For example, asking someone to complete a multiple-choice test on bicycle riding would not be as valid as watching someone ride, or fail to ride, a bike. It's the same in the classroom. Teachers and observers need to make sure that the data they gather are actually measuring what students are supposed to be learning.
Sometimes observers must settle for less reliable data in order to get more valid data. For example, data that can be gathered reliably, such as time on task, can tell us whether students look engaged, but they don’t tell us what is going on in students’ minds. A student who looks like she is thinking about a writing task might actually be thinking, “Wouldn’t it be cool if Taylor Swift moved in next door?” To get a better understanding of how students are thinking, teachers might find it more helpful to ask students to self-report on their level of engagement than using time on task, even though we cannot know for sure how accurately students are reporting.
Similarly, different types of assessments are more valid for some kinds of learning than others. Giving students quizzes or tests might be valid for measuring whether students have acquired knowledge, for example, but assessing how well students transfer skills might be more validly measured through rubrics. All data are imperfect; what matters is that we gather data that give us the most useful insight into what we are assessing.
Source: From (pp. 21–27), by J. Knight and M. Faggella-Luby, 2024, ASCD/One Fine Bird Press. Copyright 2024 by Instructional Coaching Group, LLC. Reprinted with permission.